Seasonality Patterns within the Disaster Hedge Portfolios
Constructing upon the established analysis on market seasonality and the potential for front-running to spice up related earnings, this text investigates the appliance of seasonal methods inside the context of disaster hedge portfolios. Not like conventional asset allocation methods that will falter throughout market stress, disaster hedge portfolios are designed to supply draw back safety. We look at whether or not incorporating seasonal timing into these portfolios can improve their efficiency and return-to-risk ratios, doubtlessly providing superior risk-adjusted returns in comparison with static or non-seasonal approaches.
Crucially, we additionally analyze the extent to which the advantages of seasonal timing are diminished by the actions of different market members looking for to take advantage of the identical predictable patterns. This analysis contributes to the prevailing literature by specializing in the intersection of seasonality and disaster hedging, offering precious insights for traders looking for to optimize portfolio resilience in turbulent market environments.
Background
The existence of seasonality in monetary markets has been a subject of educational inquiry for many years. Early research, akin to Keim (1983), documented predictable patterns in inventory returns throughout months and quarters. Theobald (1992) additionally explored these patterns, notably in thinly traded shares. More moderen analysis by Asness et al. (2013) confirms the presence of seasonal results, highlighting their potential worth in portfolio building.
Whereas seasonality gives alternatives for enhanced returns, it’s also nicely understood that traders exploit these patterns, resulting in potential front-running, elaborated by Moskowitz and Grinblatt (2002), the place seasonality-driven methods underperform attributable to arbitrage exercise. In response to our research, Entrance-Working Seasonality in US Inventory Sectors, a front-running technique that selects sectors ETFs primarily based on their efficiency within the earlier month, can outperform the benchmark. This means potential seasonality in US inventory sectors.
Formulation in Context of Current Analysis & This Examine Assertion
Constructing on this basis, this paper investigates the efficacy of incorporating seasonality into disaster hedge portfolios. We look at whether or not seasonal components can enhance portfolio efficiency throughout market downturns and whether or not front-running conduct boosts or mitigates such advantages.
Our evaluation thus extends the prevailing literature hole on seasonality in conventional asset allocation by focusing particularly on disaster hedge portfolios, providing precious insights for portfolio managers looking for to navigate tough market circumstances.
Hedging Funding Universe Asset Choice
We intention to validate the seasonal patterns inside a specified cross-hedge portfolio. To perform this Black Swan Hedging Mannequin of Gioele (2019) from the Antifragile Asset Allocation technique, we adopted the hedge portfolio constituents delineated in Desk 5‘s listing of ETFs. We’re intently analyzing our hedging utility in regards to the introduced hedge portfolio.
Following Giordano‘s footsteps, we additionally nod to Nassim Nicholas Taleb, choices dealer turned educational researcher, for his authentic considering that produced the conceptualization of Black Swans and Antifragility.
Following within the desk, six chosen property are listed:
For instance, the information may be obtained from Yahoo Finance to elucidate the funding universe additional. Nevertheless, lately, Yahoo Finance discontinued free end-of-day knowledge downloads. Consequently, we suggest sourcing knowledge from EODHD.com – the sponsor of our weblog. EODHD gives seamless entry to +30 years of historic costs and elementary knowledge for shares, ETFs, foreign exchange, and cryptocurrencies throughout 60+ exchanges, obtainable by way of API or no-code add-ons for Excel and Google Sheets. As a particular provide, our weblog readers can take pleasure in an unique 30% low cost on premium EODHD plans. The evaluation covers the interval from 2007-02-13 to 2024-09-05, and the day by day granularity is enough for the kinds of research carried out.
As our benchmark, probably the most diversified and sophisticated composition of property is feasible inside the strategies’ realm.
Seasonality Sorts
Moreover, we are able to now transfer on to describing the 2 seasonality sorts employed on this research:
Time-series seasonality (TSS)—Just like our commodities research, we conduct an intra-asset comparability, analyzing efficiency over 12 months and analyzing months t-12 (true-seasonality) and t-11 (front-running seasonality) for potential predictors.
Cross-sectional seasonality (CSS): We carry out an inter-asset comparability inside the portfolio, figuring out the highest and backside performers inside teams. Once more, we concentrate on months t-12 and t-11 as predictors.
Time-Sequence Seasonality (TSS)
At first, we carried out one of these seasonality to get a glimpse of each true (pure) seasonality and potential front-running elements. Moreover, the charts and tables present the efficiency of the benchmark, which is the equally-weighted portfolio constructed from the six ETFs current within the disaster hedge funding universe.
First, we present the ends in graphical type, appreciating the funding quantity within the fairness curve.
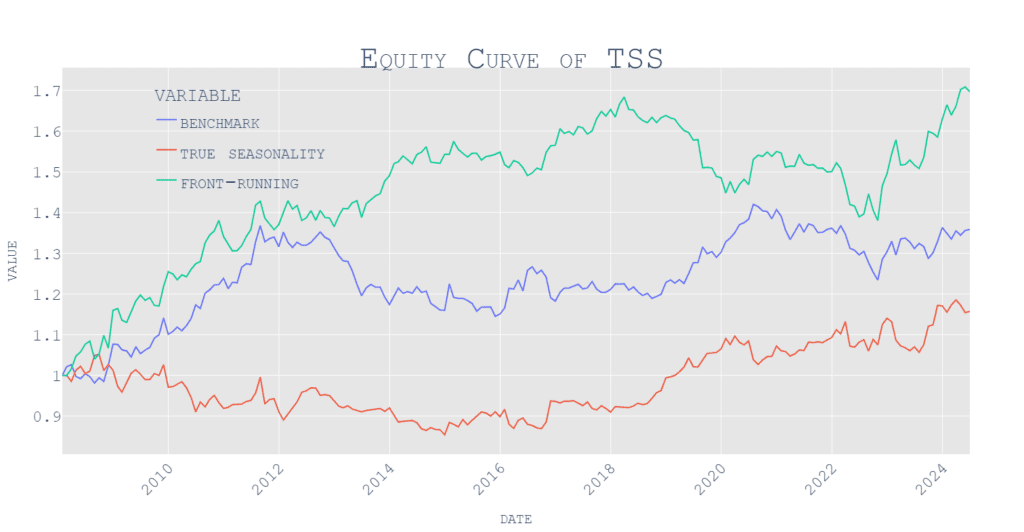
Once more, presenting vital efficiency and threat metrics within the type of a desk:


Apparently sufficient, selecting any methodology would yield optimistic efficiency. Nevertheless, as prior to now articles/papers associated to the seasonality patterns, we see that the efficiency of the seasonal technique, which selects ETFs primarily based on their efficiency over the identical month (previous January predicts future January returns, and many others. and many others.) underperforms benchmark (equally-weighted portfolio of ETFs) and various technique that front-runs the seasonal sign by one month (previous January predicts the efficiency of the December returns, and many others. and many others.)
Now, allow us to strive the second strategy…
Cross-Sectional Seasonality (CSS)
Right here, we are going to apply two variants,
long-only and
unfold (long-short) portfolios.
Entrance-Working Cross-Sectional Seasonality Methods
Figures for long-only portfolios will first be introduced, adopted by a variety top-bottom (winner-minus-loser WML) portfolio for a hard and fast variety of devices (starting from 1 to three for lengthy legs and 0 to three for brief legs). The benchmark for front-running is as soon as once more the equally weighted universe of underlying ETFs.


Here’s a returns and threat desk overview for all introduced variants:

Surprisingly, all variants of the front-running technique outperform the benchmark. The long-only variants have, on common, higher efficiency and return-risk ratios than long-short variants. The candy spot goes lengthy two property with one of the best efficiency within the month T-11. Including brief legs introduces an excessive amount of threat into the general technique.
True (Pure) Cross-Sectional Seasonality Methods
The benchmark for the true (pure) seasonality methods is once more the equally weighted portfolio of ETFs (as in earlier instances). The identical process is carried out as within the earlier part; nevertheless, this time, we go lengthy the ETFs with one of the best efficiency in T-12 month (and, moreover, brief the worst performing ETFs within the case of long-short methods):


And accompanying efficiency outcomes and threat metrics desk:

The general outcomes are disappointing when judging in opposition to front-running methods. The entire variants of the true/pure seasonal methods (primarily based on T-12 sorting) underperform front-running seasonal methods (T-11). As soon as once more, we are able to verify that even within the funding universe, which consists of ETFs that may be thought-about “disaster hedges”, front-running seasonality alerts by one month outperform different various seasonality methods.
In our disaster hedge ETF universe, front-running is clear in cross-sectional seasonal patterns and time-series seasonality. This twin prevalence underscores the pervasive nature of front-running throughout completely different dimensions of market seasonality. Incorporating seasonality into disaster hedge portfolios can considerably improve efficiency and our outcomes point out that each time-series and cross-sectional seasonal methods present sturdy draw back safety and superior risk-adjusted returns in comparison with static or non-seasonal approaches. This analysis bridges the hole between conventional asset allocation and seasonal methods, offering a pivotal framework for portfolio managers aiming to boost resilience in risky market environments.
Creator: Cyril Dujava, Quant Analyst, Quantpedia
Are you in search of extra methods to examine? Join our e-newsletter or go to our Weblog or Screener.
Do you need to be taught extra about Quantpedia Premium service? Examine how Quantpedia works, our mission and Premium pricing provide.
Do you need to be taught extra about Quantpedia Professional service? Examine its description, watch movies, overview reporting capabilities and go to our pricing provide.
Are you in search of historic knowledge or backtesting platforms? Examine our listing of Algo Buying and selling Reductions.
Or comply with us on:
Fb Group, Fb Web page, Twitter, Linkedin, Medium or Youtube
Share onLinkedInTwitterFacebookConfer with a pal